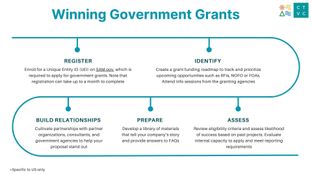
🌎 Get ready with me: Government grants edition 💅💵
A founder’s guide to winning non-dilutive funding with Elemental Excelerator
We sat down with Himanshu Gupta, CEO and founder of ClimateAI to discuss the potential of applying AI to climate issues – and where AI is overhyped.
We sat down with Himanshu Gupta, CEO and founder of ClimateAI to discuss the potential of applying AI to climate issues – and where AI is overhyped. ClimateAI brings climate forecasting and agronomics into the age of machine learning to address food system resiliency and ultimately increase farmer profitability.
Take it from the top! What should our readers know about ClimateAI?
ClimateAI offers a product called “Resiliency as a Service” in agriculture. Why agriculture? Last year alone, 25% of global climate disasters costs were absorbed by agriculture – which 70%+ of the population depends on for their livelihoods. Agricultural producers’ and suppliers’ profits are already marginal, before absorbing the majority of climate disaster costs. At ClimateAI, we saw this injustice as a great social and economic opportunity.
The agricultural market is inefficient in not rewarding resiliency. Farmers’ crop production output is measured in yield, safety, and quality. Climate change impacts all three of these. The market, however, treats a grower with more resilient output the same as their neighbor whose output may be high during a good year but will drastically suffer during a drought year.
Food companies want more reliable and quality supply chains, but don’t reward resilient producers. Growers all pay the same lending and insurance costs and all get the same contract price with the food companies. As far as public policy is concerned, the solutions to resiliency problems have only been reactive “bandaids” such as loan forgiveness programs after a climate disaster wreaks someone’s harvest. We’re building a “Resiliency as a Service” platform that allows markets to correct for inefficiencies and fund resilience in the supply chain.
What are the biggest opportunities to apply AI to climate?
I see 3 arenas where AI can make a difference to climate challenges: 1) fundamental science, 2) applied science, and 3) metrics production.
In fundamental science, AI improves the parameterization of climate models. As this work develops, we’ll see a lot less uncertainty in predictions like those from the IPCC.
ClimateAI focuses on the second bucket of applied science AI. We’re deploying AI to learn the underlying physics of climate interactions and predict extreme climate risks. The challenge is that observational data for predicting climate risks dates back only to the 1920s – a super limited dataset in the scheme of global climate changes. We’re taking an approach similar to the autonomous driving industry; if there isn’t enough data to train your algorithms on the road, you train them on a simulation. That’s ClimateAI’s approach.
We also operate in the third arena, producing industry-relevant metrics, which combines the fundamental and applied science datasets to provide business insights. Farmers have questions like: When should I plant my seeds? When should I harvest? What crops should I plant over the next 10-20 years in this location? When will climate change impact me? There’s a lot of general talk about climate change altering crop yield decades down the line, but ClimateAI understands when and to what magnitude that impact will happen.
Who are your customers and how do they use your technology?
Our customers are typically seed companies, processors, and food companies. We don’t directly sell to farmers, who walk the challenging fine line of narrow profit margins. Our customers deploy our solutions to farmers as part of their value added services, and through that we’re able to scale at a much faster rate.
Our customers use our technology for production planning. What should I think about next season? Will I be able to meet sales demand? This is all based on the ability to predict extreme weather in your location.
Another set of our customers use our technology to predict where crops will move in the future. Would that increase the risk of deforestation? Where is the best place to plant for optimal soil health?
These are our current two agricultural products. We also have API solutions to predict climate risk which can be applied in the water and energy sectors.
How would customers in the energy or water space use this precision AI tool?
While we’re primarily focused on the agriculture sector, it’s inherently linked with the water and energy industries. For example, let’s say that you’re an asset manager of a hydropower plant. Your region’s seasonal temperature drives the level of river water flow. A sudden heat wave in February causes accelerated snow melt in the spring, causing the summer season to be fraught with drought. In this scenario, we can pinpoint your plant’s peak energy production in March and forecast suboptimal production in the summer, coincidently when energy prices and demand are at their highest.
Our technology can also be used by the energy sector to predict solar and wind droughts – similar to water droughts. What kind of system shock is there if the northeastern corridor of the US (which is the most wind-intensive) experiences a “wind drought” for 6 hours?
To help companies and industries to understand how AI applied to climate data affects them, I find myself referencing a three-pronged framework: (1) What is the hazard (e.g., drought, flood, or wildfire)? (2) What is the company’s exposure? (3) Where is the vulnerability? These three questions delineate the sectoral impact and determine decisions that stakeholders should be making.
Have you turned down potential clients due to lack of alignment with your climate-first mandate?
Yes. Our actions will always be climate positive, so we’ll never work with the fossil fuel industry. We’ve turned down a couple of customers from the oil and gas industry already.
How has your background in climate policy been helpful in understanding how to apply AI to climate?
When I was in the policy sphere in the Government of India and the U.S, I analyzed climate change from a macro-level bird’s-eye view – looking at what kind of policy inflection points could accelerate the deployment of low carbon tech within the industry. We also rolled out big data initiatives across various industries in India for researchers to conduct analysis on it. In this process, I was able to understand what the pain points were and where technology could make a dent – at a micro-level approach. Now, I’m analyzing climate with both the macro and micro-lenses in trying to understand the value of AI and improved data in addressing this issue.
Where is AI overhyped – particularly as it relates to climate?
AI can be overkill. A simple linear regression can create enough customer value, rather than applying complex neural networks to the problem.
If not agriculture, where else would you apply AI within climate?
There’s a lot of AI work needed to create weather and climate data. As an example, high resolution global datasets for even very simple variables like precipitation are rarely available. This is where AI could be the most helpful.
Curious to learn more about ClimateAI? Their website and Himanshu’s Medium are good sources of more thinking. Likewise, ClimateAI is always open to interacting with bright minds passionate about the climate space. Keep tuned to their jobs page for upcoming Product Marketing Manager and Operations Lead job descriptions, and in the interim email them directly for opportunities to collaborate.
Interested in more content like this? Subscribe to our weekly newsletter on Climate Tech below!
A founder’s guide to winning non-dilutive funding with Elemental Excelerator
Infrastructure investing for impact with Banyan Infrastructure's Amanda Li
Venturing into nature with Diego Saez-Gil at Pachama